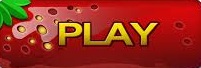

10 developed a hierarchical statistical mechanical modeling (HSM) approach to predict the interactions between peptides and protein binding domains (PBDs).

More recently, the booming deep-learning technologies have provided feasible solutions to model protein–ligand or protein–protein interactions (PPI) with better accuracy while requiring less computational resources. Unfortunately, solving such 3D structures is generally time-consuming and expensive 1, letting alone consuming a large number of computational resources due to the high computational complexity of the energy functions.
FRAMEWORK PROTEIN SCAFFOLD FREE
Most of these docking approaches require three-dimensional (3D) structure information to calculate binding free energies. There are many well-established docking strategies for determining PepPIs, which can be roughly divided into local (e.g., DynaRock 6 and Rosseta FlexPepDock 7) and global docking methods (e.g., PIPER-FlexPepDock 8 and HPEPDOCK 9) according to the extent of input structural information. Structure-based methods such as molecular docking inherently tackle the problem by modeling structural poses at atom level and predicting binding affinities. In addition, these approaches are not able to identify crucial binding residues, which hits a roadblock in deciphering the underlying mechanisms of PepPIs. These methods often require known protein–ligand interactions as supervised labels and pairwise similarity scores of proteins (or ligands) as input features, which is often impractical for large-scale data owing to the huge computational complexity of similarity calculation. For example, CGKronRLS 4 and NRLMF 5 calculate sequence similarities and then use machine-learning models to predict interactions between proteins and their ligands. Sequence-based methods mainly exploit primary sequence information to model the interactions. Sequence-based methods and structure-based methods are two mainstream approaches for protein–ligand interaction prediction. To mitigate this issue, a number of computational methods have been developed to facilitate peptide drug discovery. Despite this fact, it is generally time-consuming and costly to determine pepPIs experimentally 1, 3. Owing to their safety, favorable tolerability profiles in human bodies, and good balance between flexibility and conformational rigidity, peptides have become good starting points for the design of novel therapeutics, and identifying accurate peptide–protein interactions (PepPIs) is crucial for the invention of such therapeutics. Peptides play crucial roles in human physiology by interacting with a variety of proteins and participating in many cellular processes, such as programmed cell death, gene expression regulation, and signal transduction 1, 2. CAMP can serve as a useful tool in peptide-protein interaction prediction and identification of important binding residues in the peptides, which can thus facilitate the peptide drug discovery process. In addition, CAMP outperformed other state-of-the-art methods on binary peptide-protein interaction prediction. Comprehensive evaluation demonstrated that CAMP can successfully capture the binary interactions between peptides and proteins and identify the binding residues along the peptides involved in the interactions. Here, we present a deep learning framework for multi-level peptide-protein interaction prediction, called CAMP, including binary peptide-protein interaction prediction and corresponding peptide binding residue identification. However, most of the existing prediction approaches heavily depend on high-resolution structure data. Recently, a number of computational methods have been developed to predict peptide-protein interactions. Peptide-protein interactions are involved in various fundamental cellular functions and their identification is crucial for designing efficacious peptide therapeutics.
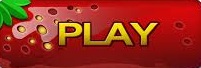